Stefan Balke, Matthias Dorfer, Luis Carvalho, Andreas Arzt, Gerhard Widmer
Abstract
Connecting large libraries of digitized audio recordings to their corresponding sheet music images has long been a motivation for researchers to develop new cross-modal retrieval systems. In recent years, retrieval systems based on embedding space learning with deep neural networks got a step closer to fulfilling this vision. However, global and local tempo deviations in the music recordings still require careful tuning of the amount of temporal context given to the system. In this paper, we address this problem by introducing an additional soft-attention mechanism on the audio input. Quantitative and qualitative results on synthesized piano data indicate that this attention increases the robustness of the retrieval system by focusing on different parts of the input representation based on the tempo of the audio. Encouraged by these results, we argue for the potential of attention models as a very general tool for many MIR tasks.
Cross-Modal Embedding Space
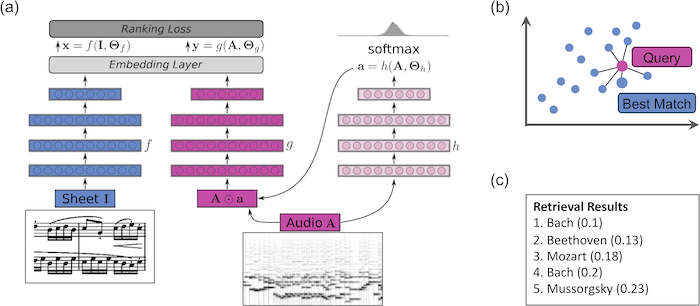
Examples
Johann André - Sonatine
L. v. Beethoven - Piano Sonata (Op. 79, 1st Mvt.)
Mussorgsky - Pictures at an Exhibition VIII: Catacombae
Citation
@inproceedings{BalkeDCAW19_SoftAttRetrieval_ISMIR, author = {Stefan Balke, Matthias Dorfer, Luis Carvalho, Andreas Arzt, Gerhard Widmer}, title = {Learning Soft-Attention Models for Tempo-invariant Audio-Sheet Music Retrieval}, booktitle = {Proceedings of the International Society for Music Information Retrieval Conference ({ISMIR})}, pages = {573-–580}, address = {Delft, The Netherlands}, year = {2019} }